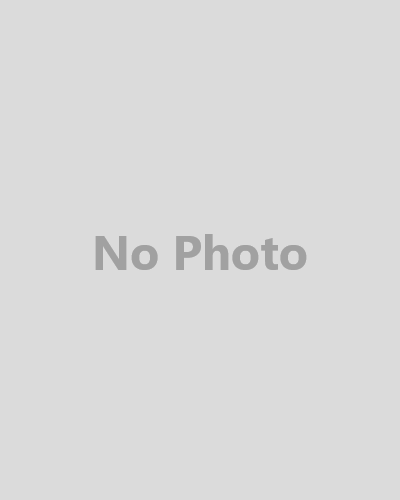
特徴量の順序は人工ニューラルネットワークモデルの性能に影響するか? - 日本のオンライン調査に基づく統合失調症有無の分類 -
医学部・公衆衛生学
Does the Feature Order Affect the Performance of Artificial Neural Network Model?
A classifier for the existence of schizophrenia based on a Japanese online survey
Introduction
In epidemiology studies, the features are collected regardless of the order. Usually, the participants fill the questionnaire with fixed structure or get health checkup. The content of the answers and health checkup results will be considered not the order of them.
Regarding machine learning, it is not clear if the order of features affects the performance or not. Indeed, suppose there are 76 features collected, namely there are 76! different orders of the features. The model constructed by using the training set will be different for each order of the features.
In this study, we will explore the effect of feature orders on the performance of the machine learning model. Since 76! is too big to be reviewed, we will start with 10 thousand permutations of the feature order.
Methods
Data were collected from an internet research agency’s pooled panel (Rakuten Insight, Inc.). In brief, we sampled subjects aged 20-75 years who currently have schizophrenia (SZ, n = 223) and those who do not currently have SZ (health control, n = 1776).
Answers to the question items in online survey were formatted to one response variable (the existence of SZ, “yes” or ”no”) and 75 feature variables (demographic, health-related backgrounds, physical comorbidities, psychiatric comorbidities, and social comorbidities). Details of the study participants and variable definition are described elsewhere. (Matsunaga, 2023)
Classifier
The classifier is trained using a 5-hidden-layer artificial neural network (ANN, Figure1). Compared with the classic linear models, ANN has merits on dealing with large scaled data and mining out more potential information.
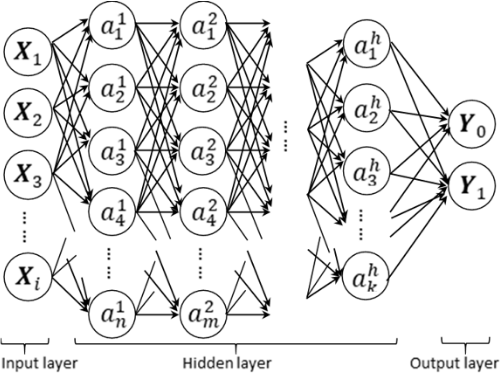
a refers to neurons in hidden layers.
Y refers to response variables during training process and prediction results during test process.
Experiment processing
As described before, considering 76! orders are too big to be reviewed, 10,000 different orders were randomly generated, and we separate the sample into training set and test set. The order of features for all individuals will be rearranged following one of the orders. The model will be trained by using training set, and tested with test set, the area under the receiver operating characteristic curve (AUC) results will be recorded and we repeat the experiment for each order among the 10,000 order types. (Figure 2) The following thres hold are used to evaluate the performance of AUC score: 0.5 = no discrimination; 0.5-0.7 = poor discrimi-nation; 0.7-0.8 = acceptable discrimina-tion; 0.8-0.9 = excellent discrimination; >0.9 = outstanding discrimination.
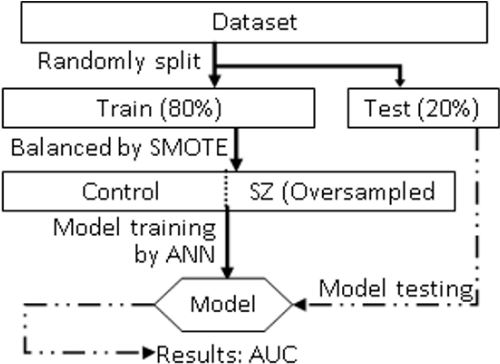
Results
The distribution of the AUC scores from the 10,000 experiments is shown in Figure 3. The AUC score of the vast majority of models is around 0.9, which has achieved excellent discrimination. The AUC score of a few models is between 0.5 and 0.7, and the AUC score of a very small number of models is higher than 0.95, which has achieved outstanding discrimination.
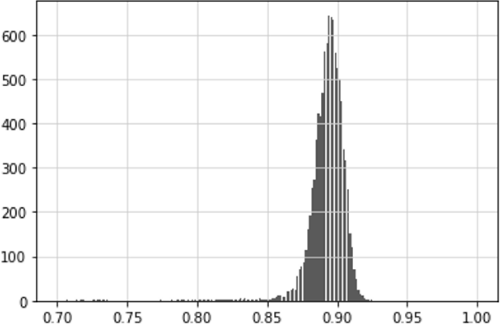
Discussion
The order of features is likely to have an impact on the performance of the ANN model.
We hypothesize that if there is a correlation between features and responses, there exists an optimal feature order that can enable the model to achieve its highest performance. The optimal feature order may help to elucidate the relationship among the features as well as the associations between the features and the outcome.
Limitations
Currently, the number of trials is insufficient. We will increase it in the next stage of the experiment. To test the generalizability of our conclusions, we will expand the trials to multiple datasets. We need to further explore whether a unique optimal feature order exists.
シーズ&ニーズ
複数のデータセットで実験をテストするため、他の部署・講座と協力することを期待しています。また、人工ニューラルネットワークを含む機械学習手法をさらなる疫学研究に適用することを目指しています。
This study was supported by the Health and Labour Sciences Research grant from the MHLW, Japan (JPMH21GC1018 to AO), Grants-in-Aid for Scientific Research from JSPS (22K21186 to YH), and FHU若手研究費 (2126 to YH).